The machine takes high resolution scans of field crops, helping to develop the next generation of ag sensors
MARICOPA, Ariz. — A massive robotic crop scanner at the Maricopa Agricultural Center takes extremely detailed measurements of crop plots and is helping to develop the next generation of agricultural sensors.
“This is meant to be a reference system for very high resolution, high quality data sets that can then be down scaled and compared to lower cost instruments that might be more cost effective going forward,” said Maria Newcomb of the University of Arizona’s School of Plant Sciences.
The sensors are located in a white steel box mounted on a 30-ton steel gantry that runs on rails that were originally 218 metres long before another 170 metres of rail were added after funding was obtained from the Bill and Melinda Gates Foundation for studies of grain sorghum.
Read Also
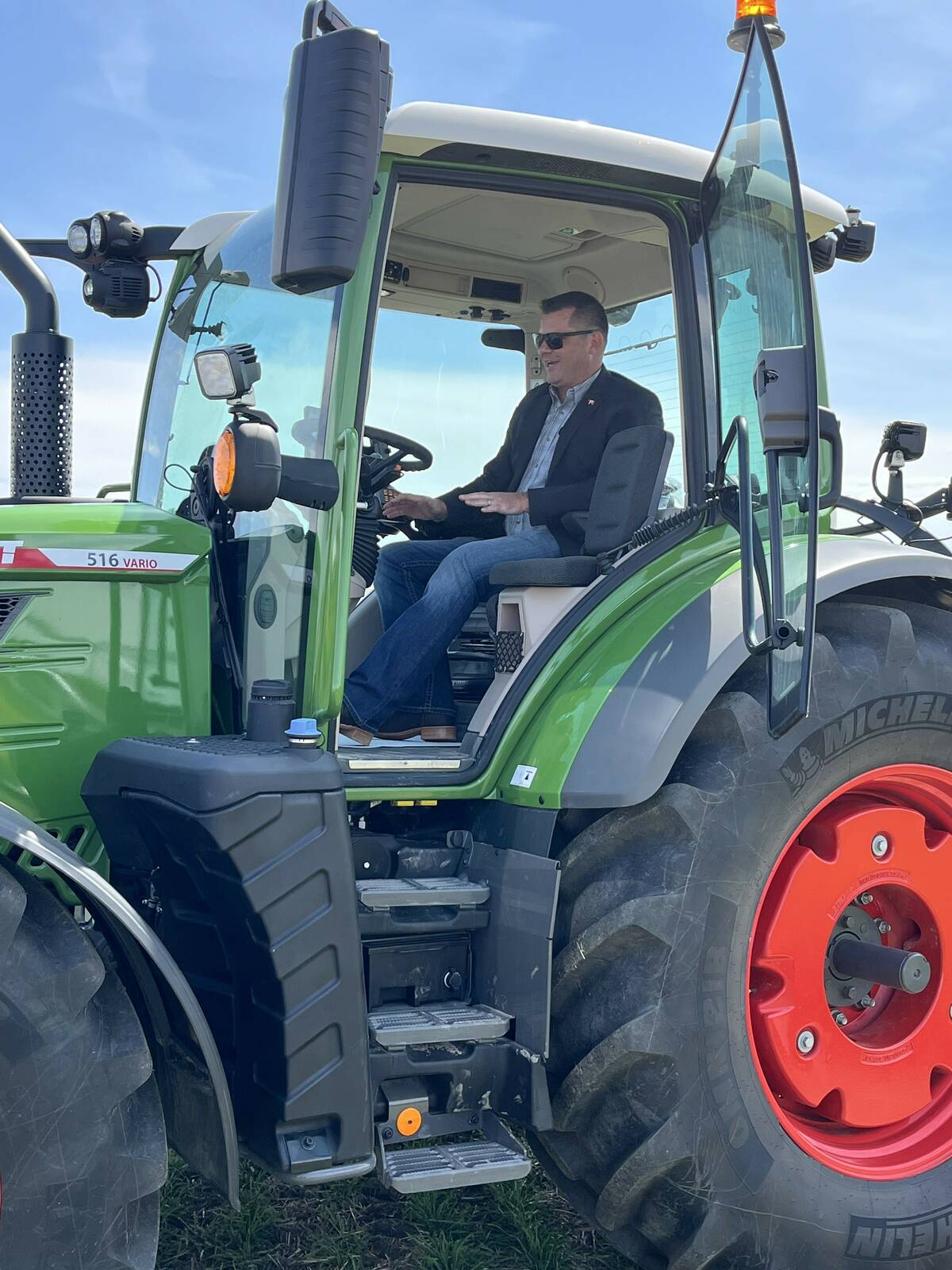
Farming Smarter receives financial boost from Alberta government for potato research
Farming Smarter near Lethbridge got a boost to its research equipment, thanks to the Alberta government’s increase in funding for research associations.
The scanner collects information on slightly less than two acres of crop plots and is able to move in three dimensions and at different velocities, depending on the research objectives and the resolution tradeoffs.
The sensor box contains two hyperspectral cameras that cover the full range of short-wave infrared (SWIR) and very-near infrared (VNIR) bands.
“It’s the VNIR instrument that generates the largest volume of data by far. It’s very high resolution data,” Newcomb said.
There is also a FLIR thermal camera for canopy temperature traits and two laser scanners capable of a one millimetre resolution.
“Even the little tiny seedlings that are emerging showed up in the point cloud data, and that’s with it operational at 3.5 metres above the little seedlings, so very high resolution,” Newcomb said.
Two RGB cameras are installed in stereo, enabling researchers to do stereo reconstruction with the RGB data.
The system also features a unique instrument for taking chlorophyll fluorescence measurements that the researchers call the PS2. It operates at night for dark-adapted plants, taking measurements of photosynthesis parameters.
“It’s fairly common to have this kind of camera in a growth chamber or a controlled environment, but there are not very many imaging field cameras like this,” Newcomb said.
“It’s also common to take measurements with hand-held instruments, but this gets a metre or so field of view, so it’s a canopy level measurement rather than leaf level.”
The information from the scans is complied in a publicly accessible repository within 48 hours so that researchers and plant breeders can have quick access to the data.
Twelve institutions are contributing to the projects, including the National Center for Supercomputing Applications (NCSA) at the University of Illinois, where the data is stored.
A fibre optic line runs from the Maricopa Agricultural Center to the NCSA to transmit the data.
“We have the potential to generate very high volumes of data, several terabytes a day when it’s using all of the instruments,” Newcomb said.
“All of that data is moved to the super computer centre in the University of Illinois and it’s their challenge to organize it and associate it with the metadata, and make it publicly available.”
The primary purpose of the crop plot scanner is to study hundreds of concurrent plot trials in which large sets of germless are tested in a field environment.
The sensors provide phenotyping from seedling emergence to as long as the experiments run.
They enable “high throughput phenotyping to match the high throughput genotyping data that came like 10 years ago or more, when that cost came way down and the volume of genetic markers that can be obtained has increased,” Newcomb said.
“Characterising the plant, the phenotyping, is the slow part of the process. It wasn’t automated and using sensors. It’s people out taking measurements in the field, which is slow and it takes a lot of people.”
Many of the crop plots are currently involved in a drought study, which will help direct further breeding initiatives.
“We know which of those 240 varieties have the same chromosomal segments that seem to be the markers, or the triggers or the definers of how do you react when you’re running out of water,” she said.
“What we want is a marker of that so that we can work at a scale that allows us to drill down to the genes and eventually to direct it.”
She said the ability to find genetic features of plant varieties that enable comparisons of genotype to phenotype has been the bottleneck when it comes to variety developments.
“Some of our collaborators are using machine learning algorithms for the image analysis to take all of that high volume data and turn it into plant traits, like leaf width, estimates of biomass, canopy cover, canopy temperature.”
She said an important part of the project is the work on as many as 100 spectral indices from the hyperspectral data that is still being explored in the data analysis.
Rick Ward, a professor in the university’s School of Plant Sciences, is studying the use of drone sensors by comparing their readings to the large crop-scanner sensors.
“In two weeks I could fly this field twice. It takes 15 minutes each time, about an hour in between, and the correlation between the spectral indices for the 504 or 700 plots out here, would be almost one,” Ward said.
“So for an individual breeder, which is my background, to walk this and take notes, on anything, just to see all 700 would take a couple of hours. To do it twice, or to have two people do it twice, say, scoring a biomass or greenness, the correlation between the two values would be low.”
Beyond the technical repeatability of finding similar scan results on the same crop plot, testing at the facility has shown it is possible to differentiate between different varieties of the same crop type with the sensors.
“When we have three replications of 300 some genotypes, you get the average of the three plots for each genotype, and an hour later I get almost a correlation of one, so that means it’s biologically and genetically relevant,” Ward said.
“And when we do genotype to phenotype association, we find very small chromosomal regions that affiliate with the high end, the middle and the low end of spectral characteristics, and with fairly low resolution drones.”
He said hyperspectral sensors, which collects and processes information from across the electromagnetic spectrum, are showing significant promise in the research.
“They’re able to discriminate between every variety in a field, that has extraordinary implications from a breeding perspective.”
By referring the crop scanner data to data from drone sensors, it becomes possible to downscale the data to find how narrow of a sensor band is required to answer research questions.
The sensor data being compiled at the Maricopa Agricultural Center is helping to develop sensor parameters best suited for specific agricultural applications, and then other sensors can be developed to meet those parameters. For instance, there can be specific parameters for issues such as crop stress caused by drought, disease or pests.
“Can you fly at 40 metres or 60 metres? The best way to do those experiments is to get an extraordinarily high level of resolution and then randomly reduce the precision,” Ward said.
“Basically remove pixels or increase overlap between pixels and then that gives you performance characteristics, whether it’s for a tractor base that informs digital agriculture, or it’s satellites, planes, drones and the sensors and the equipment that goes along with them.”